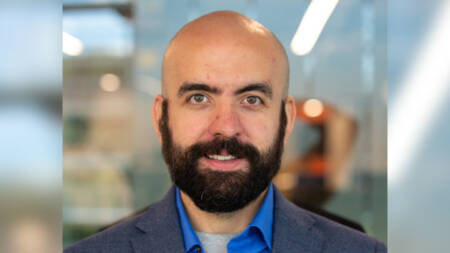
2:30 pm to 3:30 pm
1403 Tepper School Building
Abstract:
Dexterous tool manipulation is a dance between tool motion, deformation, and force transmission choreographed by the robot’s end-effector. Take for example the use of a spatula. How should the robot reason jointly over the tool’s geometry and forces imparted to the environment through vision and touch? In this talk, I will present our recent progress on touch-centric approaches to dexterous tool manipulation: multimodal compliant tool representations via neural implicit representations and our recent progress on tactile control with high-resolution and highly deformable tactile sensors. Our methods seek to address two fundamental challenges in object manipulation. First, the frictional interactions between these objects and their environment is governed by complex non-linear mechanics, making it challenging to model and control their behavior. Second, perception of these objects is challenging due to both self-occlusions and occlusions that occur at the contact location (e.g., when wiping a table with a sponge, the contact is occluded). We will demonstrate how implicit functions can seamlessly integrate with robotic sensing modalities to produce high-quality tool deformation and contact patches and how high-resolution tactile controllers can enable robust tool-use behavior despite the complex dynamics induced by the sensor mechanical substrate. We’ll conclude the talk by discussing future directions for dexterous tool-use.
Bio:
Nima Fazeli is an Assistant Professor of Robotics at the University of Michigan (2020-Present) and affiliate Faculty of Computer Science and Engineering (CSE) in EECS and Mechanical Engineering at UM. Nima is also the director of the Manipulation and Machine Intelligence (MMint) Lab. Nima’s primary research interest is enabling intelligent and dexterous robotic manipulation with emphasis on the tight integration of mechanics, perception, controls, learning, and planning. Nima received his PhD from MIT (2019) and completed his postdoctoral training (2020) working with Prof. Alberto Rodriguez. He received his MSc from the University of Maryland at College Park (2014) where he spent most of his time developing models of the human (and, on occasion, swine) arterial tree for cardiovascular disease, diabetes, and cancer diagnoses. His research has been supported by the NSF CAREER, National Robotics Initiative, and Advanced Manufacturing, the Rohsenow Fellowship and featured in outlets such as The New York Times, CBS, CNN, and the BBC.