
RI Seminar
April
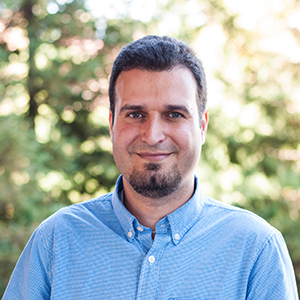
3:30 pm to 4:30 pm
1305 Newell Simon Hall
Recent advances in Deep Reinforcement Learning (DRL) algorithms provided us with the possibility of adding intelligence to robots. Recently, we have been applying a variety of DRL algorithms to the tasks that modern control theory may not be able to solve. We observed intriguing creativity from robots when they are constrained in reaching a certain goal. To introduce the topic, I will talk about some of the experiments that are being done to show the capabilities and limitations of modern Deep Reinforcement Learning approaches, including those of sparse rewards and continuous observations and action spaces. An in depth explanation of how Hindsight Experience Replay (HER) has been used to obtain dense results from sparse environments when using Deep Deterministic Policy Gradient (DDPG) agents will be given. I will then show how we have modified some of these experiments to have a deeper understanding of the intelligence we are developing, and what are the baseline environmental characteristics that make the robots achieve higher levels of creativity during their problem solving scenarios.
Bio:
Professor Farimani joined the Department of Mechanical Engineering at Carnegie Mellon University in the fall of 2018. He was previously a postdoctoral fellow at Stanford University. He received his PhD in Mechanical Engineering in 2015.
His lab at CMU focuses on the problems at the interface of Mechanical Engineering, datascience and machine learning. His lab uses the state of the art deep learning and machine learning algorithms and tools to learn, infer and predict the physical phenomena pertinent to mechanical engineering.
He received the Stanley I. Weiss best thesis award from the University of Illinois in 2016 and was recognized as an Outstanding Graduate Student in 2015. During his post-doctoral fellowship at Stanford, Dr. Barati Farimani has developed data-driven, deep learning techniques for inferring, modeling, and simulating the physics of transport phenomena and for materials discovery for energy harvesting applications.