
RI Seminar
October
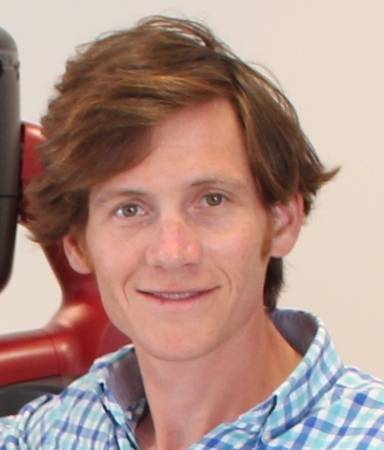
3:30 pm to 4:30 pm
1305 Newell Simon Hall
Abstract: Advances in perception have been integral to transitioning robots from machines restricted to factory automation to autonomous agents that operate robustly in unstructured environments. As our surrogates, robots enable people to explore the deepest depths of the ocean and distant regions of space, making discoveries that would otherwise be impossible. The age of robots operating in isolation is giving way to a new generation of agile robots that work in close collaboration with people. As our partners, robots will improve our quality of life in revolutionary ways—they will empower people with physical impairments to maintain their independence, provide the elderly with cognitive and physical care, and improve the efficiency and agility of manufacturing and logistics. Achieving this promise demands robots that people can efficiently command and naturally interact with. I will present methods that treat language as a novel “sensing” modality, enabling robots to leverage the flexibility and expressivity of natural language to communicate with and learn from their human partners. I will first describe an algorithm for language understanding in a priori unknown environments that exploits information implicit and explicit in the user’s utterance to learn rich distributions over the latent spatial and semantic properties of the scene. A learned belief space policy then reasons over these distributions to infer suitable navigation and manipulation behaviors. Next, I will describe a related approach that leverages language as a form of weak supervision to learn manipulation models from narrated demonstrations. I will conclude by presenting a framework that enables robots to succinctly convey information to their human partners through natural language generation.
Brief Bio: I am an assistant professor and director of the Robot Intelligence through Perception Laboratory (RIPL) at the Toyota Technological Institute at Chicago (TTI-Chicago), a philanthropically endowed academic computer science institute located on the University of Chicago campus. I also hold a part-time faculty appointment in the Department of Computer Science at the University of Chicago. Prior to joining TTI-Chicago, I was a research scientist in the Computer Science and Artificial Intelligence Laboratory (CSAIL) at the Massachusetts Institute of Technology (MIT), where I was fortunate to work with Seth Teller. I received my PhD from the Joint Program between MIT and the Woods Hole Oceanographic Institution, under the supervision of John Leonard. My thesis considered the problem of scaling robotic mapping and localization to larger unknown environments. I studied feature-based algorithms for simultaneous localization and mapping (SLAM) whereby a robot builds a map of the world while concurrently estimating its position in the map. My thesis work proposed a sparse information filter algorithm that is scalable and also preserves estimate consistency. The approach maintains a Gaussian probability distribution over the robot and map states, and takes advantage of insights into the natural structure of this model for SLAM. The Exactly Sparse Extended Information Filter (ESEIF) exploits a sparse parametrization of this distribution to reduce the computational and memory costs from quadratic to linear in the map’s size. In addition to the gains in efficiency, a primary contribution of the algorithm is its ability to achieve sparsity in a principled, yet simple way that preserves consistency. For more information, please see the IJRR paper or my thesis.
Host: Howie Choset
For an appointment, please email: Peggy Martin (pm1e@andrew.cmu.edu)
In person COVID restrictions will be enforced