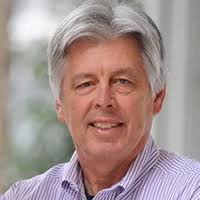
3:30 pm to 4:30 pm
1305 Newell Simon Hall
Abstract: In this talk, we will describe methods to enable robots to grasp novel objects using multi-modal data and machine. The starting point is an architecture to enable robotic grasp planning via shape completion using a single occluded depth view of objects. Shape completion is accomplished through the use of a 3D CNN. The network is trained on our open source dataset of over 440,000 3D exemplars captured from varying viewpoints. At runtime, a pointcloud captured from a single point of view is fed into the CNN, which fills in the occluded regions of the scene, allowing grasps to be planned and executed on the completed object, which extends to novel objects as well. We have extended this network to incorporate both depth and tactile information. Offline, the network is provided with both simulated depth and tactile information and trained to predict the object’s geometry, thus filling in regions of occlusion. At runtime, the network is provided a partial view of an object and exploratory tactile information is acquired to augment the captured depth information. We demonstrate that even small amounts of additional tactile information can be incredibly helpful in reasoning about object geometry. We also provide experimental results comparing grasping success using our method.
Biosketch: Peter Allen is Professor of Computer Science at Columbia University, and Director of the Columbia Robotics Lab. He is the recipient of the CBS Foundation Fellowship, Army Research Office fellowship, the Rubinoff Award for innovative uses of computers, and the NSF PYI award. His current research interests include robotic grasping, medical robotics and Brain-Computer Interfaces for Human-Robot interaction.
Host: Matt Mason
For Appointments: Jean Harpley (js3g@andrew.cmu.edu)