
RI Seminar
February
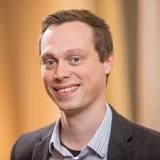
3:30 pm to 4:30 pm
Abstract: Before learning robots can be deployed in the real world, it is critical that probabilistic guarantees can be made about the safety and performance of such systems. In recent years, safe reinforcement learning algorithms have enjoyed success in application areas with high-quality models and plentiful data, but robotics remains a challenging domain for scaling up such approaches. Furthermore, very little work has been done on the even more difficult problem of safe imitation learning, in which the demonstrator’s reward function is not known. This talk focuses on new developments in three key areas for scaling safe learning to robotics: (1) a theory of safe imitation learning; (2) scalable reward inference in the absence of models; (3) efficient off-policy policy evaluation. The proposed algorithms offer a blend of safety and practicality, making a significant step towards safe robot learning with modest amounts of real-world data.
Brief Bio: Scott Niekum is an Assistant Professor and the director of the Personal Autonomous Robotics Lab (PeARL) in the Department of Computer Science at UT Austin. He is also a core faculty member in the interdepartmental robotics group at UT. Prior to joining UT Austin, Scott was a postdoctoral research fellow at the Carnegie Mellon Robotics Institute and received his Ph.D. from the Department of Computer Science at the University of Massachusetts Amherst. His research interests include imitation learning, reinforcement learning, and robotic manipulation. Scott is a recipient of the 2018 NSF CAREER Award and 2019 AFOSR Young Investigator Award.
Host: Oliver Kroemer
For Appointments: Stephanie Matvey (smatvey@andrew.cmu.edu)