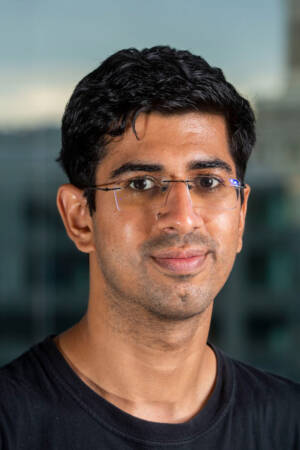
3:30 pm to 4:30 pm
1305 Newell Simon Hall
Abstract: The world we live in is incredibly diverse, comprising of over 10k natural and man-made object categories. While the computer vision community has made impressive progress in classifying images from such diverse categories, the state-of-the-art 3D prediction systems are still limited to merely tens of object classes. A key reason for this stark difference is the relative difficulty of acquiring supervision — while it is easy to annotate a semantic label for an image, obtaining ground-truth 3D for learning at scale is infeasible. But do we really need such ground-truth 3D for learning? In this talk, I will present a learning-based approach that can train from unstructured image collections, using only segmentation outputs from off-the-shelf recognition systems as supervisory signal, thus allowing us to scale to an order of magnitude more classes than existing works. I will also show how continually (instead of independently) learning 3D inference for new classes can further improve performance. Finally, while single-view image collections allow us to learn coarse category-level 3D inference, I will also show how sparse multi-view collections can allow us to infer fine instance-level 3D shapes for generic objects using as few as 8 images.
Bio: Shubham Tulsiani is an incoming Assistant Professor in the CMU School of Computer Science. Prior to this, he was research scientist at Facebook AI Research (FAIR). He received a PhD. in Computer Science from UC Berkeley in 2018. He is interested in building perception systems that can infer the spatial and physical structure of the world they observe.
Host: David Held
Point of Contact: Stephanie Matvey (snatvey@andrew.cmu.edu)