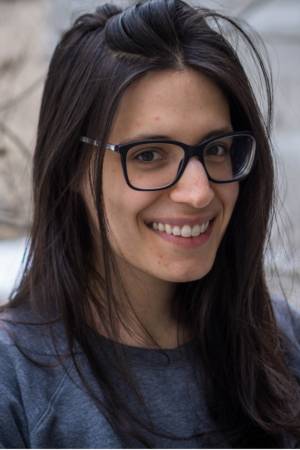
Abstract: Robotic cave exploration has the potential to create significant societal impact through facilitating search and rescue, in the fight against antibiotic resistance (science), and via mapping (survey). But many state-of-the-art approaches for active perception and autonomy in subterranean environments rely on disparate perceptual pipelines (e.g., pose estimation, occupancy modeling, hazard detection) that process the same underlying sensor data in different ways leading to redundancy, inefficiency, and increased system complexity. In this talk, I will discuss recent work in leveraging generative models to unify these disparate pipelines for efficient exploration. The techniques yield gains in terms of accuracy in pose estimation and occupancy modeling as compared to the state of the art. Further, the compactness of the generative model produces benefits in communication efficiency, which is critical for bandwidth-constrained multi-robot or human-robot operations. Broader opportunities for active perception and future investigations for evolving, improving, and expanding the application of the representation will be discussed.
Brief Bio: Wennie Tabib is a Systems Scientist in the Robotics Institute at Carnegie Mellon University. She received a B.S. degree in computer science in 2012, M.S. degree in robotics in 2014, and Ph.D. degree in computer science in 2019 from Carnegie Mellon University. She researches perception, planning, and learning algorithms to enable safe autonomy in significantly three-dimensional, complex environments. Her graduate research was supported by a Paul and Daisy Soros Fellowship for New Americans and NASA Space Technology Research Fellowship.
Host: Sebastian Scherer
Point of Contact: Stephanie Matvey (smatvey@andrew.cmu.edu)