
PhD Thesis Proposal
May
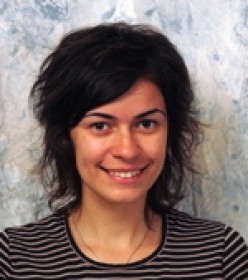
10:00 am to 12:00 am
Event Location: GHC 8102
Abstract: The goal of this thesis is to enable robots to produce motion that is suitable for human-robot collaboration and co-existence. Most motion in robotics is purely functional: industrial robots move to package parts, vacuuming robots move to suck dust, and personal robots move to clean up a dirty table. This type of motion is ideal when the robot is performing a task in isolation. Collaboration, however, does not happen in isolation. In collaboration, the robot’s motion has an observer, watching and interpreting the motion.
In this work, we move beyond functional motion, and introduce the notion of an observer into motion planning, so that robots can generate motion that is mindful of how it will be interpreted by a human collaborator. We formalize predictability and legibility as properties of motion that naturally arise from the inferences that the observer makes, drawing on action interpretation theory in psychology. We propose models for these inferences based on the principle of rational action, and use a combination of constrained trajectory optimization and machine learning techniques to enable robots to plan motion that is predictable or legible.
Finally, we evaluate the impact of such motion on two paradigms of collaboration: performing a task in a shared workspace, and performing a task by sharing the robot’s autonomy. We expect that predictability and legibility will not only increase task performance, but also make the collaboration process more seamless, increasing trust and perceived robot capability, and decreasing cognitive load on the human collaborator.
Committee:Siddhartha Srinivasa, Chair
Geoffrey Gordon
Jodi Forlizzi
Henrik Christensen, Georgia Institute of Technology