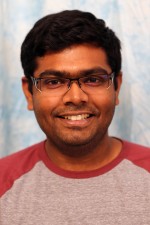
Carnegie Mellon University
3:00 pm to 4:00 pm
Abstract
Robotics and artificial intelligence have witnessed tremendous progress in the past decade. Yet, we are still far from building the general purpose robot butler that can autonomously operate in homes and help with manipulation tasks like household chores. Grasping is an important action primitive for manipulation and needs to generalize to unstructured settings. This thesis aims to demonstrate that current data-driven techniques are not yet ready for grasping-in-the-wild and proposes frameworks to bridge this gap.
In completed work, we demonstrate three approaches to overcome this gap. First, most existing policies are trained on datasets collected in curated settings (in lab or simulation) and hence may not cope with the mismatch in data distribution when deployed in unstructured visual environments like people’s homes. We build and open-source a low-cost mobile manipulator platform to parallelize data collection in homes and show that policies trained on this data generalize to novel objects in unseen homes. Second, most grasping methods focus on bin-picking clutter or 3-DOF planar grasping. We present a 6-DOF grasp generation approach for structured clutter which is more typical in the kitchen and warehouses. Third, we present a self-supervised tactile-based re-grasping framework to close the loop for grasping.
The proposed work will focus on purposeful grasping, which takes grasp synthesis a step further to reason about post-grasp utility. We will explore how 1) prior symbolic knowledge from humans can be used to generalize semantic grasping and 2) learning continuous grasp manifolds could improve trajectory optimization over the current paradigm of sampling motion-agnostic discrete grasp sets.
Thesis Committee Members
Abhinav Gupta, Chair
Oliver Kroemer
David Held
Dieter Fox, University of Washington
Sonia Chernova, Georgia Institute of Technology