
PhD Speaking Qualifier
July
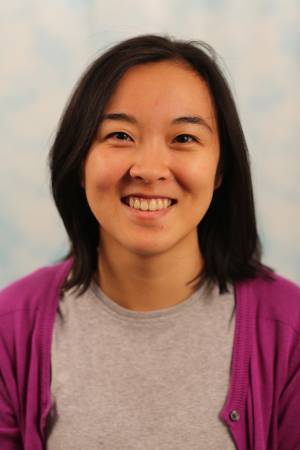
Abstract:
In theory, control barrier functions (CBFs) provide a convenient means to construct provably safe controllers. However, a typical problem is that the constructed controller will exceed input limits, and merely clipping the inputs will break all safety guarantees. To address this practical flaw, we consider synthesizing a CBF that will respect input limits. We pose this as a min-max optimization problem. Our key ideas are to use a generic neural CBF and to train it using an efficient learner-critic framework. Unlike previous synthesis techniques, ours can scale to large, nonlinear systems. We demonstrate this on a 10D state, 4D input problem of balancing a pendulum on a quadrotor. For this problem, our trained CBF respects input limits almost 100% of the time vs. just 80% for a standard baseline.
Committee:
Prof. John Dolan
Prof. Changliu Liu
Prof. David Held
Jaskaran Grover