
PhD Speaking Qualifier
March
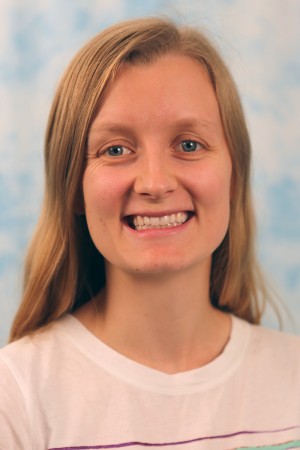
Abstract:
Exploration is one of the core challenges in reinforcement learning. A common formulation of curiosity-driven exploration uses the difference between the real future and the future predicted by a learned model. However, predicting the future is an inherently difficult task which can be ill-posed in the face of stochasticity. In this work, we introduce an alternative form of curiosity that rewards novel associations between different senses. Our approach exploits multiple modalities to provide a stronger signal for more efficient exploration. Our method is inspired by the fact that, for humans, both sight and sound play a critical role in exploration. We present results on several Atari environments and Habitat (a photorealistic navigation simulator), showing the benefits of using an audio-visual association model for intrinsically guiding learning agents in the absence of external rewards.
Committee:
Abhinav Gupta (advisor)
David Held
Ruslan Salakhutdinov
Brian Okorn