
VASC Seminar
February
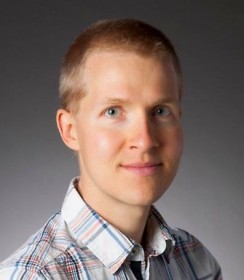
3:00 pm to 4:00 pm
Event Location: NSH 1507
Bio: Damien Teney recently joined Carnegie Mellon University as a post-doctoral researcher with a fellowship of the Belgian-American Educational Foundation. His research interests are at the intersection of computer vision, machine learning, and robotics. He is currently working with Martial Hebert, focusing on visual scene understanding and semantic segmentation. He previously worked on topics such as video segmentation, motion analysis, object recognition and pose estimation for robotic grasping. He was previously affiliated with the University of Bath (UK, 2013, Prof. Matthew Brown) and the Intelligent and Interactive Systems at the University of Innsbruck (Austria, 2011-2013, Prof. Justus Piater). He is originally from Belgium, where he obtained his M.Sc. (2009) and PhD (2013) in Computer Science at the University of Liège.
Abstract: In video segmentation, motion can be a powerful cue to disambiguate appearance and distinguish distinct objects. Identifying motion is however not trivial in natural scenes, where complex phenomena can arise, for example a dissipating cloud of smoke, or flickering reflections on water. This talk will expand on the challenges faced by traditional methods for optical flow and video segmentation, and I will present solutions to characterize such dynamic textures and segment them. Firstly, we will see how to design spatiotemporal filters, to be convolved with the video to capture texture and motion cues. Such 3D filters are similarly applicable to simple translations, complex non-rigid or even transparent motions, and provide additional features to a classical segmentation framework. Secondly, I will present an extension to the graph-based hierarchical segmentation algorithm to incorporate supervision, to improve the separation between the objects/textures of interest, using ground truth segmentations or semantically-labeled examples.