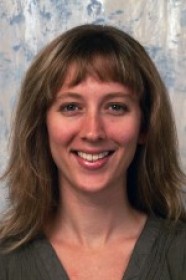
1:00 pm to 12:00 am
Event Location: NSH 1305
Abstract: Humans and robots would benefit from having rich semantic maps of the terrain in which they operate. Mobile robots equipped with sensors and perception software could build such maps as they navigate through a new environment. This information could then be used by humans or robots for better localization and path planning, as well as a variety of other tasks. However, it is hard to build good semantic maps without a great deal of human effort and robot time. Others have addressed this problem, but they don’t provide a high level of semantic richness, and in some cases their approaches require extensive human data labeling and robot driving time.
We propose to use a combination of better sensors and features, both proprioceptive and exteroceptive, and self-supervised learning to solve this problem. We will enhance proprioception by exploring the use of new sensing modalities such as sound and vibration, and in turn we will increase the number and variety of terrain types that can be estimated. The proprioceptive features will then be used to teach exteroceptive features coming from camera and range data how to estimate those same terrain types more reliably through self-supervised learning schemes. The exteroceptive features will be used to spatially register the terrain estimates into a larger map of the surrounding environment. Our claim is that self-supervised learning will make the exteroception more reliable since it can be automatically retrained for new locations without human supervision. We will conduct experiments to support this claim by collecting extensive data sets and then comparing classification accuracies, training set sizes, and map sizes. We will explore a variety of feature extraction techniques, both from proprioceptive and exteroceptive data, and we will explore learning techniques that can be used to build self-supervised frameworks.
Committee:Anthony Stentz, Chair
Martial Hebert
David Wettergreen
Larry Matthies, Jet Propulsion Laboratory