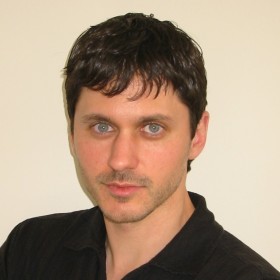
3:30 pm to 12:00 am
Event Location: NSH 1305
Bio: Karl Iagnemma is a principal research scientist in the Mechanical Engineering department at the Massachusetts Institute of Technology, and director of the Robotic Mobility Group. He holds a B.S. from the University of Michigan, and an M.S. and Ph.D. from MIT, where he was a National Science Foundation Graduate Fellow. He has performed postdoctoral research at MIT, and has been a visiting researcher at the NASA Jet Propulsion Laboratory and the National Technical University of Athens (Greece). Dr. Iagnemma’s primary research interests are in the areas of design, sensing, motion planning, and control of mobile robots in outdoor terrain, including modeling and analysis of robot-terrain interaction. He is author of the monograph “Mobile Robots in Rough Terrain: Estimation, Planning and Control with Application to Planetary Rovers” (Springer, 2004). He has recently led research programs for agencies including the U.S. Army TARDEC, the Army Research Office, DARPA, the NASA Mars Program Office, Ford Motor Company, and the NASA Institute for Advanced Concepts, among others. He has authored or co-authored many conference and journal papers on a wide range of robotic topics, and has consulted for various private companies and government agencies including the Army and the Jet Propulsion Laboratory.
Abstract: Recent traffic safety reports from the National Highway Traffic and Safety Administration show that in 2008 alone, over 37,000 people were killed and another 2.3 million injured in motor vehicle accidents in the United States. The longstanding presence of passive safety systems in motor vehicles, combined with the ever-increasing influence of active systems, has contributed to a decline in these numbers from previous years. Still, the need for improved active hazard avoidance technologies remains significant.
In this presentation, I will discuss progress in the development of a novel passenger vehicle hazard avoidance framework that semi-autonomously shares control with a human driver while maintaining stability, preserving controllability, and avoiding hazards. This framework leverages the predictive and constraint-handling capabilities of Model Predictive Control to iteratively re-plan an optimal trajectory through the drivable region of the environment, assess the threat this trajectory poses to the vehicle, and regulate driver and controller inputs to maintain that threat below a specified threshold. The control framework will be described, simulation results discussed, and the presentation will conclude with experimental results obtained on a semi-autonomously-controlled Jaguar S-Type.