
PhD Thesis Proposal
May
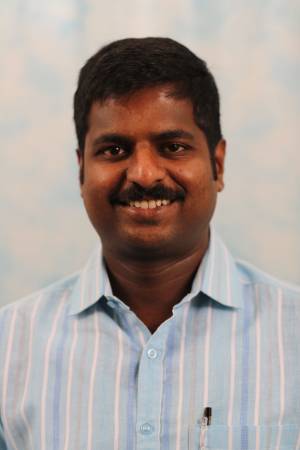
Carnegie Mellon University
Abstract:
Spectral mapping is an essential task in exploration as it expands our understanding of material composition in an explored region. Although imaging spectrometers are ideal for obtaining spectra to construct spectral maps, their large size, high power consumption, and operational complexity make them impractical for small rovers and limited missions. In contrast, RGB cameras are lightweight, energy-efficient, and commonly used for essential tasks, such as hazard detection and terrain characterization.
Our work proposes a novel method that utilizes data from RGB cameras for spectral mapping. We accomplish this by representing spectra in a latent space and learning a mapping from RGB to this latent space. Despite the lower dimensionality of RGB cameras, which have only three channels compared to hundreds of channels from a hyperspectral camera, we demonstrate that a regression framework can be established by using just a few hyperspectral measurements for learning. This framework can be used to construct spectral maps with high accuracy. Furthermore, we show that our approach is flexible and can be extended to accommodate sensors with more channels as they become available. To validate our methodology, we tested our methods in Cuprite, a mineral-rich site in Nevada.
Our proposed work encompasses three primary objectives. Firstly, we aim to leverage the high-spatial resolution of RGB camera images to extract supplementary information, including relative elevation, texture (2D), and roughness (3D), to augment the accuracy of spectral maps. Secondly, we intend to develop a latent network that can exhibit consistent performance on a diverse range of datasets. Finally, we aim to demonstrate the viability of this approach on additional sites to showcase its versatility and potential for broader applications.
Thesis Committee Members:
David Wettergreen, Chair
George Kantor
Michael Kaess
Roger Clark, Planetary Science Institute