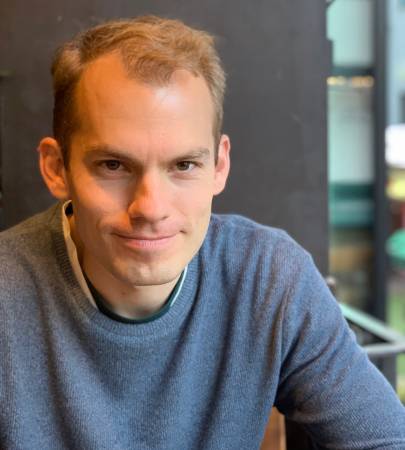
3:30 pm to 4:30 pm
1305 Newell Simon Hall
Abstract:
We are entering an era of highly general AI, enabled by supervised models of
the Internet. However, it remains an open question how intelligence emerged in
the first place, before there was an Internet to imitate. Understanding the
emergence of skillful behavior, without expert data to imitate, has been a
longstanding goal of reinforcement learning (RL), but the majority of previous
work has been strikingly narrow in scope (e.g., controlling a single robot for a
specific manipulation task). In this talk I will share some of our group’s recent
work toward generalist RL agents. I will cover 1) quasimetric RL, which
employs geometric structures inherent in decision-making problems to expedite
multi-task learning, 2) incorporating latent variables into agent policies to
generate a greater diversity of behaviors, and 3) developing scalable
environments that support more open-ended tasks.
Bio:
Phillip Isola is the Class of 1948 Career Development associate professor in
EECS at MIT. He studies computer vision, machine learning, and AI. He
completed his Ph.D. in Brain & Cognitive Sciences at MIT, and has since spent
time at UC Berkeley, OpenAI, and Google Research. His research has been
recognized by the PAMI Young Researcher Award, a Packard Fellowship, and
a Sloan Fellowship, among other awards. His current research focuses on
trying to scientifically understand human-like intelligence.