
PhD Speaking Qualifier
December
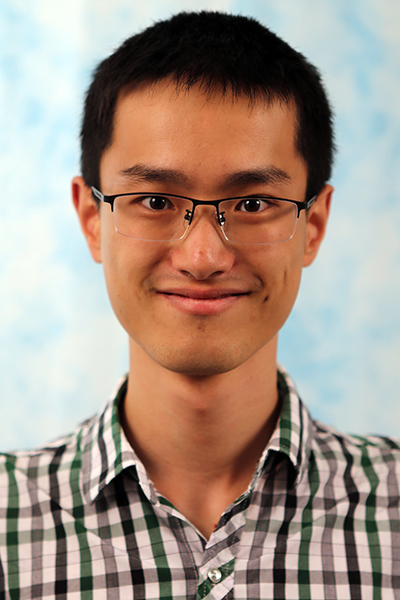
Abstract:
Imagine walking along a busy sidewalk, do you track the movement of every single individual? Or do you simply group pedestrians with similar moving patterns and then track the movement of this group? Grouping is a common behavior in pedestrian navigation and it is typically inappropriate for a robot to cut through the social space within pedestrian groups. In this talk, I will present our approaches to model social group shapes and then predict group splits and merges based on the evolution of such social group shapes. Predicting group splits and merges also benefits social navigation by empowering the robot to foresee opening or closing gaps among groups. We demonstrate our deep learning based approach’s effectiveness by comparing it against creative interpretations of the state-of-the-art trajectory prediction models. We also show that our model captures interesting features from social group shape evolutions. From this project, we identified weaknesses in datasets and group space modeling. We then describe our ongoing efforts in data collection and personal space modeling with promising results at the end of our presentation.
Committee:
Aaron Steinfeld (Advisor)
Kris Kitani
Jean Oh
Ishani Chatterjee