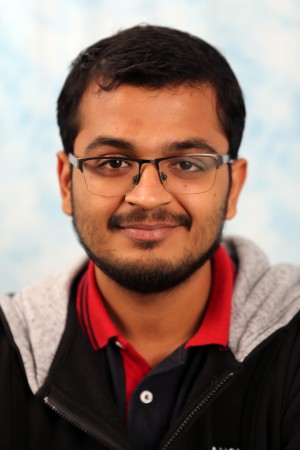
Carnegie Mellon University
3:30 pm to 4:30 pm
Zoom link: https://cmu.zoom.us/j/3388909661
Title: On-Policy Reinforcement Learning for Learning to Drive in Urban Settings
Abstract:
Traditional autonomous vehicle pipelines that follow a modular approach have been very successful in the past both in academia and industry, which has led to autonomy deployed on road. Though this approach provides ease of interpretation, its generalizability to unseen environments is limited and hand-engineering of numerous parameters is required, especially in the prediction and planning systems. Recently, Deep Reinforcement Learning has been shown to learn complex strategic games and perform challenging robotic tasks, which provides an appealing framework for learning to drive.
In this thesis, we propose two works that formulate the urban driving tasks using reinforcement learning and learn optimal control policies primarily using waypoints and low-dimensional representations, also known as affordances. We demonstrate that our agents when trained from scratch learn the tasks of lane-following and driving around intersections as well as learn to stop in front of other actors or traffic lights even in the dense traffic setting. Further, we also propose an algorithm which we term as Exploratory Policy Search (EPS) that combines forward search with model-free reinforcement learning algorithms to find the optimal policy with increased exploration.
Committee:
Jeff Schneider (Advisor)
David Held
Benjamin Eysenbach