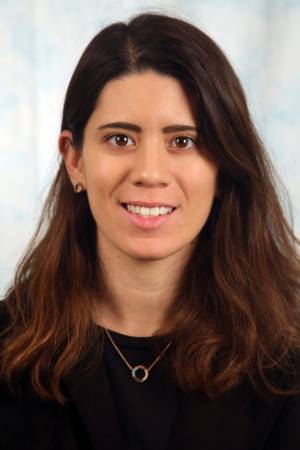
Abstract:
As autonomous robots are increasingly expected to operate in dynamic, human-centered environments, it is crucial to develop robot policies that ensure safe and seamless interactions with humans, all while allowing robots to complete their intended tasks efficiently. To achieve this, robots must be capable of making informed decisions that account for human preferences, ensuring social compliance, and adhering to safety constraints to avoid endangering humans and their surroundings.
In this context, research in fields like urban driving, social navigation, and, more recently, shared airspace, has largely focused on developing predictive models of human interactions directly from recorded data. These models aim to capture a distribution of human social preferences and safety constraints, which can then inform downstream robot decision-making policies. However, despite the growing availability of datasets, evaluation benchmarks, and modeling techniques, state-of-the-art methods remain unreliable for real-world deployment, often unable to generalize in novel environments and rare events.
Thesis Committee Members:
Jean Oh (Chair)
Sebastian Scherer
Andrea Bacjsy
Alexandre Alahi (EPFL)
Jonathan Francis (Bosch Center for AI)