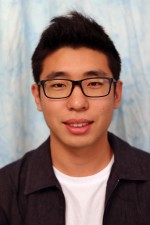
Carnegie Mellon University
2:00 pm to 3:00 pm
1305 Newell Simon Hall
Abstract
In this work, we address two current challenges in real-time visual-inertial odometry (VIO) systems – efficiency and accuracy. To this end, we present a novel approach to tightly couple visual and inertial measurements in a fixed-lag VIO framework using information sparsification. To bound computational complexity, fixed-lag smoothers perform marginalization of variables but consequently deteriorate accuracy and especially efficiency. Current state-of-the-art approaches work around this by selectively discarding measurements and marginalizing additional variables. However, such strategies are sub-optimal from an information-theoretic perspective. In contrast, our approach formulates an optimization based on Kullback-Leibler divergence to preserve most of the information. To validate our approach, we conduct extensive real-time drone tests and perform comparisons to current state-of-the-art fixed-lag VIO methods in the EuRoC visual-inertial dataset. The experimental results show that the proposed method achieves competitive and superior accuracy in almost all trials.
Speaker Bio
Shih-Chieh (Jerry) Hsiung is an M.S. student in the Robot Perception Lab at the Robotics Institute advised by Prof. Michael Kaess. He is broadly interested in the intersection of information theory, probabilistic theory and nonlinear optimization. His current research is focused on improving optimization methods in visual-inertial state estimation. Prior to CMU, Jerry received his B.S. in Computer Science from Harvey Mudd College.