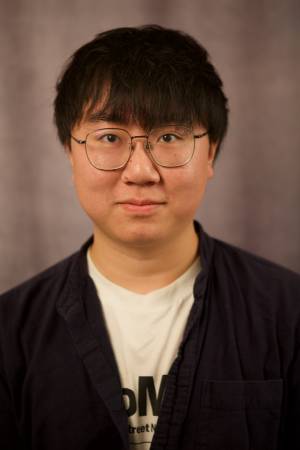
Abstract:
Denoising diffusion models have emerged as a powerful paradigm for generative modeling, which has been widely used for perception, generation, and action. These models can be utilized through sampling or score distillation; however, existing methods lack controllability in sampling and suffer from limited diversity in score distillation. In this thesis, we propose two complementary mechanisms to enhance the controllability and diversity of diffusion-based generation. First, we introduce a score rescaling approach that enables users to steer the sampling diversity of diffusion models without requiring any modifications to training. This method, validated across diverse tasks—including pose estimation, depth prediction, image generation, and robotic manipulation—demonstrates that adjusting the sampling distribution can lead to significant performance improvements. Second, we address the inherent mode-seeking limitation in score distillation for 3D optimization. Inspired by the diffusion sampling process, we propose a novel formulation that encourages optimization to follow diverse generation paths, thereby improving sample diversity while maintaining fidelity. We further introduce an approximation to adapt this formulation to practical settings where generation trajectories cannot be strictly preserved. We empirically validate our approach across multiple applications, including 2D optimization, text-to-3D generation, and single-view reconstruction. Together, these contributions advance the flexibility and effectiveness of diffusion models, broadening their applicability in generative modeling and beyond.
Committee:
Prof. Shubham Tulsiani (advisor)
Prof. Deva K. Ramanan
Prof. Jun-Yan Zhu
Sheng-Yu Wang