
MSR Thesis Defense
April
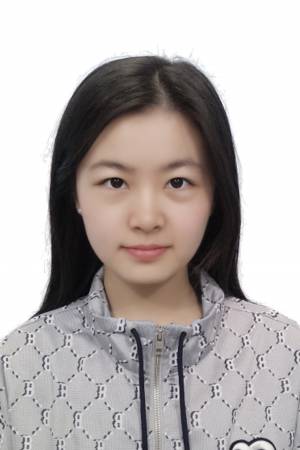
Abstract:
Accurate representation in media is known to improve the well-being of the people who consume it. There is a growing concern about the increasing use of generative AI in media as the generative image models trained on large web-crawled datasets such as LAION are known to produce images with harmful stereotypes and misrepresentations of various groups, including underrepresented cultures. It is infeasible to collect a sufficiently large dataset of representative, highly curated data to retrain a model, such as Stable Diffusion, from scratch. We improve inclusive representation in generated images by (1) engaging with communities to collect a culturally representative dataset that we call the Cross-Cultural Understanding Benchmark (CCUB) dataset and (2) proposing a novel Self-Contrastive Fine-Tuning (SCoFT) method that leverages the model’s known biases to self-improve. SCoFT is designed to prevent overfitting on small datasets, encode only high-level information from the data, and shift the generated distribution away from misrepresentations encoded in a pre-trained model. We evaluate our method with participants who are personally familiar with the cultures in the CCUB dataset. Our findings indicate that fine-tuning on CCUB decreases offensiveness and increases the cultural representation of generated images, a trend further enhanced by our proposed Self-Contrastive Fine-Tuning method. Additionally, we show that our models produce a greater diversity of generated images.
Committee:
Prof. Jean Oh (advisor)
Dr. Ji Zhang
Prof. Jun-Yan Zhu
Peter Schaldenbrand