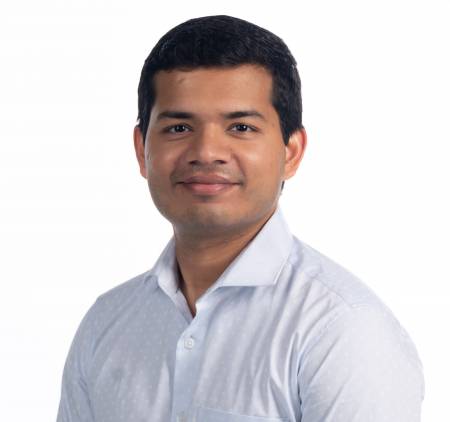
3:30 pm to 4:30 pm
Newell-Simon Hall 3305
The real world has infinite visual variation – across viewpoints, time, space, and curation. As deep visual models become ubiquitous in high-stakes applications, their ability to generalize across such variation becomes increasingly important. In this talk, I will present opportunities to improve such generalization at different stages of the ML lifecycle: first, I will discuss proactive strategies based on leveraging simulation to augment the long-tail of real training data, followed by reactive strategies based on domain adaptation to recover from unforeseen distribution shift. Finally, inspired by mutation software testing, I will present an automated framework that leverages foundation models for text and image synthesis to surface model vulnerabilities by generating language-guided counterfactual images.
Bio: Viraj Prabhu is a final year computer science Ph.D. student at the Georgia Institute of Technology, advised by Judy Hoffman. He is broadly interested in computer vision and machine learning, with a focus on developing robust AI systems that can be reliably deployed in the wild. He received his Master’s degree in Computer Science at Georgia Tech in 2019, where he was advised by Devi Parikh and awarded the MS research award. His work has been published at major AI conferences including NeurIPS, ICCV, and EMNLP, and he has served as a reviewer for CVPR, ECCV, ICCV, ICLR, NeurIPS, and ACL, twice being recognized as an outstanding reviewer (CVPR and NeurIPS 2021). He has previously held positions at NVIDIA, Salesforce, Curai, Virginia Tech, Adobe, and Google Summer of Code. He graduated from the Birla Institute of Technology and Science Pilani in 2015 with a Bachelor’s degree in Computer Science.
Homepage: www.virajprabhu.com
Sponsored in part by: Meta Reality Labs Pittsburgh