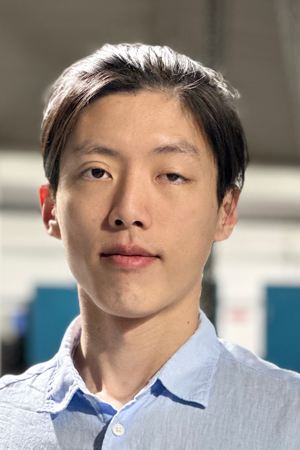
With modern robotic technologies, seafloor imageries have become more accessible to both researchers and the public. This thesis leverages deep learning and 3D vision techniques to deliver valuable information from seafloor image observations.
Despite the widespread use of deep learning and 3D vision algorithms across various fields, underwater imaging presents unique challenges, such as lack of annotations, color distortion, and inconsistent illumination, which limit the effectiveness of off-the-shelf algorithms. This thesis first develops a method to learn consistent deep features from underwater observations at different scales by introducing an algorithm based on contrastive learning. Without any annotations but geo-tags, we show that such deep features are efficient in inferring the vehicle location in a 3D reconstructed map at a small map scale. This thesis further tackles the more fundamental problem of building 3D reconstruction from raw images with heavy effects from light sources and medium interference. The following algorithms are developed to achieve seafloor 3D reconstruction with photorealistic quality: (i) Deep water true color restoration with NeRF (ii) Camera-light calibration for robotic platforms (iii) Dark environment relighting with 3DGS (iv) Recurrent underwater caustic removal.
From the real-world data we collected from robot deployments, we learned that simply enforcing consistency in geometry, appearance, and embedding does not necessarily produce accurate results. In this thesis, we develop algorithms and systems that wrap around 3D representations, which learn useful information from real-world underwater robotic data. These systems improve the ability of robots to navigate, map, and understand the environment, with zero annotations from humans.
Thesis Committee Members:
Matthew Johnson-Roberson, Chair
Matthew O’Toole
Shubham Tulsiani
Katie Skinner, University of Michigan