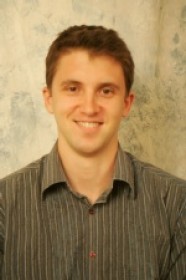
1:30 pm to 12:00 am
Event Location: Smith Hall 100
Abstract: The appearance of an outdoor scene is determined to a great extent by the prevailing illumination conditions. However, most practical computer vision applications treat illumination more as a nuisance rather than a source of signal. In this thesis proposal, we suggest that we should instead embrace illumination, even in the challenging, uncontrolled world of consumer photographs.
Our first main contribution is an understanding of natural illumination from images. This is, in general, a hard problem given the wide appearance variation in scenes. Fortunately, natural illumination, while complex, is far from being completely arbitrary. It has a structure that is well understood in atmospheric optics, but which has hardly been exploited in vision and graphics. We introduce methods for automatically estimating the illumination conditions from two types of uncontrolled outdoor image datasets: webcams and single images. The variation in sun position and sky appearance over time can be exploited to obtain viewing and illumination geometry in webcam sequences. For single images, the sky is a weak estimator of illumination and can be combined in a probabilistic way with other scene features and priors from large image collections.
Our second main contribution is to exploit the knowledge of illumination in order to synthesize novel, realistic visual content. Instead of creating appearance using the traditional computer graphics pipeline, we propose to borrow the appearance of the world that is contained in existing photo collections and webcam datasets. We also demonstrate realistic 3-D object insertion by creating plausible high-dynamic range environment maps. This can be done in image sequences, and even in single images, completely automatically.
We propose to address the following key questions. How do we design an object detector that is aware of illumination? Can this knowledge be used to improve scene understanding? We also propose to look at trends that arise when analyzing datasets of images: what can be learned about illumination from a small set of images taken at the same location? Or from a very large dataset of millions of images taken all over the world? Can we learn the correlation between certain scene appearance features and illumination? Can such features be used to simulate a change in illumination? Addressing such questions has implications in a broad range of applications including intelligent transportation, surveillance, human-robot interaction, and digital entertainment.
Committee:Alexei A. Efros, Co-chair
Srinivasa G. Narasimhan, Co-chair
Martial Hebert
Peter Belhumeur, Columbia University