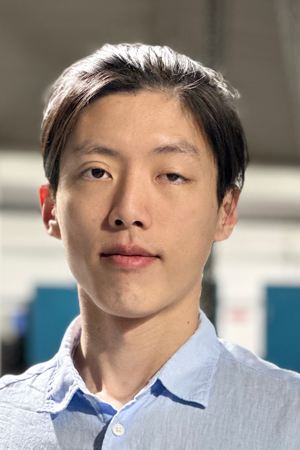
Abstract:
With modern robotic technologies, seafloor imagery has become more accessible to researchers and the public. This thesis leverages deep learning and 3D vision techniques to deliver valuable information from seafloor image observations collected by robotic platforms.
Despite the widespread use of deep learning and 3D vision algorithms across various fields, underwater imaging presents unique challenges, such as lack of annotations, color distortion, and inconsistent illumination, which limit the effectiveness of off- the-shelf algorithms. This thesis tackles the fundamental problem of building 3D representations from raw underwater images with heavy effects from light sources and medium interference. The following algorithms are developed to achieve seafloor 3D reconstruction with photorealistic quality: (i) Deep water true color restoration with NeRF (ii) Camera-light calibration for robotic platforms (iii) Dark environment re- lighting with 3DGS (iv) Recurrent underwater caustic removal. With the large amount of seafloor data as training data collected by robots, this thesis further investigates the use of deep generative models to generate large-scale underwater terrains with natural spatial variance in appearance. The synthesized terrain can be integrated with the learned underwater lighting effects, to present realistic novel-view rendering results.
This thesis shows examples of how 3D computer vision and deep generative models can be combined with physical laws, statistical principles, and foundation models, to address the unique challenges in underwater robotic perception. Collectively, these contributions lay the groundwork for reconstructing hi-fidelity underwater scenes and generating simulation environments that help close the sim-to-real gap in underwater robot perception.
Thesis Committee Members:
Matthew Johnson-Roberson (Chair)
Oliver Kroemer
Shubham Tulsiani
Matthew O’Toole
Katherine Skinner (University of Michigan)