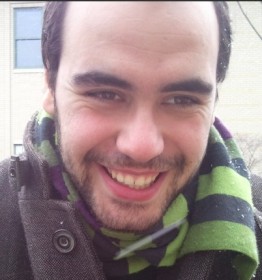
3:00 pm to 4:00 pm
Event Location: NSH 1507
Bio: Ricardo S. Cabral is working on a joint PhD program between Carnegie Mellon and IST-Lisbon. He received his Master’s degree in ECE at IST-Lisbon and a research grant from the Portuguese Science Foundation, in 2009, for work in correspondence methods for structure from motion. He received an outstanding academic achievement award in 2008 from IST-Lisbon, where he worked in several projects, including video handling for the 2012 London Olympics.
His research focuses on Computer Vision and Machine Learning, specifically in the application of low rank models for visual learning tasks. He is also a member of the Human Sensing Lab http://humansensing.cs.cmu.edu/) and of the PrintART project (http://printart.isr.ist.utl.pt), which aims to provide Artists and Art Historians with Computer Vision tools.
In the Summer of 2011, he was with the R&D Department of Industrial Light and Magic developing the next generation of special effects for the Movie industry. Last Summer, he was with Google Lightfield team researching and developing core computer vision techniques for Google Maps.
Abstract: Low rank models have been widely used for the representation of shape, appearance or motion in computer vision problems. Traditional approaches to fit low rank models make use of an explicit bilinear factorization. These approaches benefit from fast numerical methods for optimization and easy kernelization. However, they suffer from serious local minima problems depending on the loss function and the amount/type of missing data. Recently, these low-rank models have alternatively been formulated as convex problems using the nuclear norm regularizer; unlike factorization methods, their numerical solvers are slow and it is unclear how to kernelize them or to impose a rank a priori.
This paper proposes a unified approach to bilinear factorization and nuclear norm regularization, that inherits the benefits of both. We analyze the conditions under which these approaches are equivalent. Moreover, based on this analysis we propose a new optimization algorithm and a “rank continuation” strategy that outperform state-of-the-art approaches for Robust PCA, Structure from Motion and Photometric Stereo with outliers and missing data.