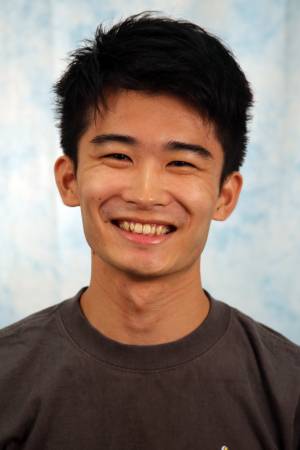
Abstract:
Visual imitation learning has shown promising results in robotic manipulation in recent years. However, its generalization to unseen objects is often limited by the size and diversity of training data. Although more large-scale robotic datasets are available, they remain significantly smaller than image and text datasets. Additionally, scaling these datasets is time-consuming and labor-intensive, making it difficult to cover a wide variety of real-world objects. To address this challenge, we explore how to incorporate prior knowledge of geometry derived from large-scale synthetic data composed of publicly available 3D model datasets to improve generalization in imitation learning without the need to expand robotic training data further.
In the first part, we frame the acquisition of a universal geometric prior as a supervised learning process applied to 3D geometry tasks. To this end, we propose two frameworks, OctMAE and ZeroGrasp, which learn the universal geometric prior through shape reconstruction and grasp pose prediction tasks. Additionally, we introduce ZeroGrasp-11B, a large-scale synthetic dataset that contains 1M RGB-D images, 12K 3D models and 11B grasps, specifically designed for training such models. Our methods achieve state-of-the-art performance on both shape reconstruction and grasp pose prediction of unseen objects on a public benchmark, demonstrating the strong capabilities of the prior. Finally, real-world pick-and-place experiments further validate its generalization to practical robotic scenarios.
Although the universal geometric prior itself demonstrates strong performance in pick-and-place tasks, robotic manipulation encompasses much more. In the second part, we focus on integrating the universal geometric prior into imitation learning to address more challenging long-horizon robotic tasks. Our proposed work primarily considers the use of the geometric prior in the latest imitation learning frameworks using diffusion models. Finally, building on the success of vision-language-action (VLA) models for language grounding and visual generalization in robotic tasks, we aim to integrate VLA models to leverage their language and visual understanding as a semantic prior. We plan to evaluate trained policies on both real and simulation setups to confirm performance improvements.
Thesis Committee Members:
Kris Kitani, Chair
David Held
Shubham Tulsiani
Sergey Zakharov, Toyota Research Institute