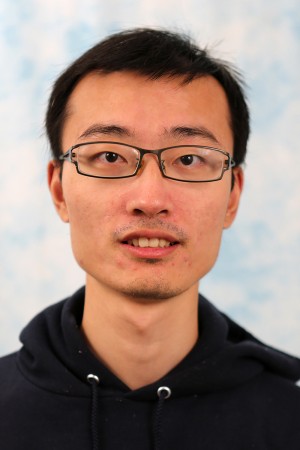
Carnegie Mellon University
Abstract:
Learning to estimate non-rigid 3D structures from 2D imaged observations is bottle-necked by the availability of abundant 3D annotated data. Learning methods that reduce the amount of required annotation is of high practical value. In this regard, Non-Rigid Structure from Motion (NRSfM) methods offer the opportunity to infer 3D structures solely from 2D annotations. Classical NRSfM approaches, however, are problematic as they rely on heuristic priors on the 3D structure (e.g. low-rank and union-of-subspace) that do not scale well to large datasets. Recently, NRSfM has seen improvement in performance by recasting the problem as an unsupervised deep learning problem. This raised fundamental questions about what novelty in NRSfM prior could the deep learning offer.
In this talk, I will advocate a 3D auto-encoder to be used explicitly as the NRSfM prior. The framework is unique as: (i) it learns the 3D auto-encoder weights solely from 2D projected measurements, and (ii) it is Procrustean in that it jointly resolves the unknown rigid pose for each shape instance. We refer to this architecture as a Procrustean Autoencoder for Unsupervised Lifting (PAUL), and demonstrate state-of-the-art performance across a number of benchmarks in comparison to recent innovations in unsupervised 2D-3D lifting.
Committee:
Simon Lucey (Advisor)
David Held
Fernando De La Torre Frade
Chen-Hsuan Lin