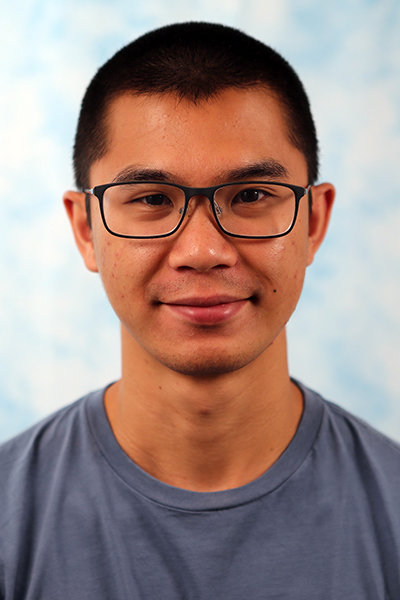
Abstract:
Advances in deep-learning based perception and maturation of volumetric RGB-D mapping algorithms have allowed autonomous robots to be deployed in increasingly complex environments. For robust operation in open-world conditions however, perceptual capabilities are still lacking. Limitations of commodity depth sensors mean that complex geometries and textures cannot be reconstructed accurately. Semantic understanding is still limited to a relatively small set of classes for which comprehensive training data is available, and segmentation predictions are subject to errors in spatial precision.
If these challenges in raw data segmentation are addressed, there is an opportunity to combine such a novel segmentation method with recent advances in neural representations. With tight coupling of segmentation and mapping approaches, there is potential for significant improvement in both tasks. In this thesis, we propose a series of approaches to more comprehensively understand raw sensor data, and better represent the environment to facilitate more accurate and rich 3D mapping.
In completed work, we propose a learning-agnostic approach for segmentation of an image sequence and point cloud representing a 3D scene. We represent scene data with a set of graphs, formulate segmentation as a graph partitioning problem, and focus on generating more precise spatial boundary predictions.
In proposed research, we first present a model aimed at generating fine-grained segmentation predictions under open-world conditions. A unified panoptic feature embedding allows for improved identification of novel classes, and an embedded graphical model facilitates precise inference. Finally, we propose to incorporate this segmentation model into a 3D mapping pipeline. As volumetric fusion based RGB-D approaches are not able to fully utilize this additional information, we propose a locally-conditioned neural representation to more efficiently and accurately represent complex geometries and textures.
Thesis Committee Members:
Michael Kaess, Chair
Deva Ramanan
Shubham Tulsiani
Joshua Mangelson, BYU