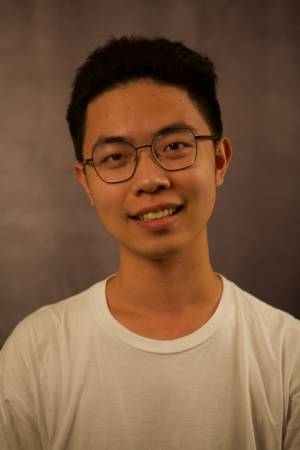
Abstract:
How can we predict future interaction trajectories of human hands in a scene given high-level colloquial task specifications in the form of natural language? In this paper, we extend the classic hand trajectory prediction task to two tasks involving explicit or implicit language queries. Our proposed tasks require extensive understanding of human daily activities and reasoning abilities about what is happening next given cues from the current scene. We also develop new benchmarks to evaluate the proposed two tasks, Vanilla Hand Prediction and Reasoning-Based Hand Prediction. We enable solving these tasks by integrating high-level world knowledge and reasoning capabilities of Vision-Language Models with the auto-regressive nature of low-level ego-centric hand trajectories. Our model, HandsOnVLM is a novel vision-language model that can generate textual responses and produce future hand trajectories through natural-language conversations. Our experiments show that HandsOnVLM outperforms existing task-specific methods and other VLM baselines on proposed tasks, and demonstrates its ability to effectively utilize world knowledge for reasoning about low-level human hand trajectories based on the provided context.
Committee:
Prof. Abhinav Gupta (advisor)
Prof. Shubham Tulsiani
Homanga Bharadhwaj