
This event has passed.
MSR Thesis Defense
April
25
Thu
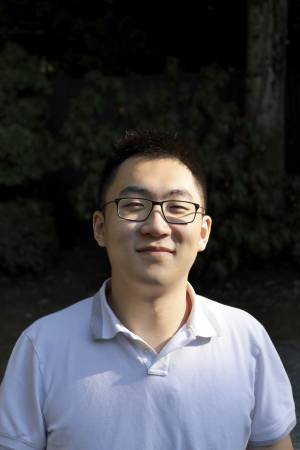
Vision Model Diagnosis and Improvement Via Large Pretrained Models
Abstract:
As AI becomes increasingly pervasive in real-world applications, the deployment of machine learning models in real-world applications has underscored critical challenges in model robustness, fairness and performance. Despite significant advances, existing models often exhibit biases, fail to generalize across diverse data distributions, and struggle with unexpected input variations, leading to suboptimal or even discrimina- tory outcomes. This thesis addresses these pressing challenges by harnessing the power of large pretrained models, especially vision generative models. In particular, two key problems are studied: (1) the identification of model biases and vulnerabilities, and (2) the utilization of synthetic data generation to improve model generalizability and performance. Along these lines, this thesis introduces two frameworks: Unsupervised Model Diagnosis (UMO) and Domain Gap Embeddings for Generative Dataset Augmentation (DoGE). The UMO framework’s ability to diagnose model vulnerabilities without extensive annotated datasets or explicit user input, combined with DoGE’s capability to augment data distributions to better align with target or underrepresented distributions, presents a powerful methodology for enhancing model fairness, robustness, and performance. Through these works, this thesis aims to enhance the robustness, fairness, and performance of machine learning models, thereby fostering the development of more reliable and equitable AI systems.
Committee:
Prof. Fernando De la Torre (advisor)
Prof. Jun-Yan Zhu
Nupur Kumari