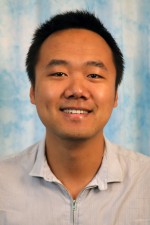
Carnegie Mellon University
2:00 pm to 3:00 pm
NSH 4305
Abstract:
Portable camera sensor systems are becoming more and more popular in computer vision applications such as autonomous driving, virtual reality, robotics manipulation and surveillance, due to the decreasing expense and size of RGB camera. Despite the compactness and portability of the small baseline vision systems, it is well-known that the uncertainty in range finding using multiple views and the sensor baselines are inversely related. For small baseline vision systems, this means high depth uncertainties even for close range objects. On the other hand, besides compactness, small baseline vision system has its unique advantages such as easier correspondence and large overlapping regions across views. How to utilize those advantages for small baseline vision setup while avoiding the limitations as much as possible? In this thesis proposal, we approach this question in terms of three aspects: scene complexity, uncertainties in the estimations and baseline distance in the setup.
We first present a method for matting and depth recovery of 3D thin structures with self-occlusions using a single-view camera with finite aperture lens. In this work, we take advantage of the small camera baselines that makes the correspondence easier. We apply the proposed method to scenes at both macro and microscales. For macro-scale, we evaluate our method on scenes with complex 3D thin structures such as tree branches and grass. For micro-scale, we apply our method to in-vivo microscopic images of micro-vessels with diameters less than 50 um.
We also utilize the small baselines for circularly placed point light sources (commonly seen in consumer devices like NESTcam, Amazon Cloudcam). We propose a two-stage near-light photometric stereo method. In the first stage, we optimizethe vertex positions using the differential images induced by small changes in lightsource position. This procedure yields a strong initial guess for the second stage that refines the estimations using the raw captured images.
To handle the estimation uncertainties inherent in the small baseline setup, we propose a learning-based method to estimate per-pixel depth and its uncertainty continuously from a monocular video stream. Compared to prior work, the proposed approach achieves more accurate and stable results, generalizes better to new datasets, and yields per-pixel depth probability map that accounts for the estimation uncertainties due to specular surface, occlusions in the scene and objects with large distance.
To deal with the subsurface light scattering in the tissue, we propose a projector-camera setup with small baseline that works in a small scale and a method that combines the approximated model for subsurface light scattering, in order to see through skins and perform in-vivo blood flow analysis on human skin.
We also propose to combine the benefits of small and large baseline vision systems, in order to handle large region occlusion and depth estimation for fine-grained structures at the same time.
Thesis Committee Members:
Srinivasa Narasimhan, Co-chair
Artur Dubrawski, Co-chair
Aswin Sankaranarayanan
Manmohan Chandraker, University of California, San Diego