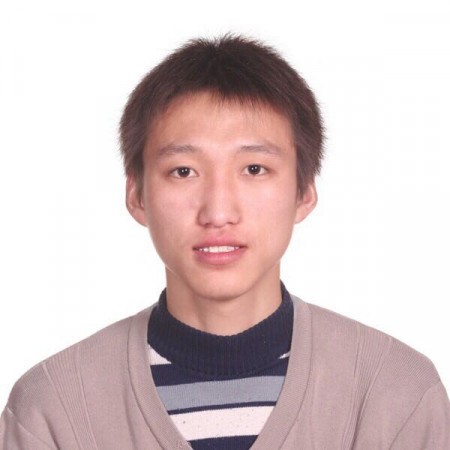
Friday, November 30
2:00 pm to 3:00 pm
3305 Newell-Simon Hall
2:00 pm to 3:00 pm
3305 Newell-Simon Hall
Visual SLAM with Semantic Scene understanding
Abstract: Simultaneous localization and mapping (SLAM) has been widely used in autonomous robots and virtual reality. It estimates the sensor motion and maps the environment at the same time. The classic sparse feature point map of visual SLAM is limited for many advanced tasks including robot navigation and interactions, which usually require a high-level understanding of 3D object and planes. Most approaches solve SLAM and scene understanding sequentially.
In this work, we propose a tightly coupled monocular object and plane SLAM to build a more accurate, meaningful and dense map. More importantly, it demonstrates that scene understanding and SLAM can improve each other in one system. To do that, we first propose an efficient 3D object detection without shape priors and graphical layout inference without box room assumptions. Then we propose a bundle adjustment system to jointly optimize camera poses with objects and planes. They can provide additional geometric, long-term scale, and semantic constraints to improve SLAM estimation. Dynamic object movements are also modeled explicitly to achieve 4D mapping and improve the estimation. Experiments on some public TUM, KITTI and collected datasets show that the proposed algorithm can get the state-of-the-art monocular camera localization accuracy and also improve the 3D object detection.
Speaker Bio: Shichao Yang is a Ph.D. student in the Mechanical Engineering at Carnegie Mellon University, advised by Prof. Sebastian Scherer in the Robotics Institute. He received a B.S in Mechanical Engineering from Shanghai Jiao Tong University in 2013. His research focuses on simultaneous localization and mapping (SLAM) and semantic scene understanding.