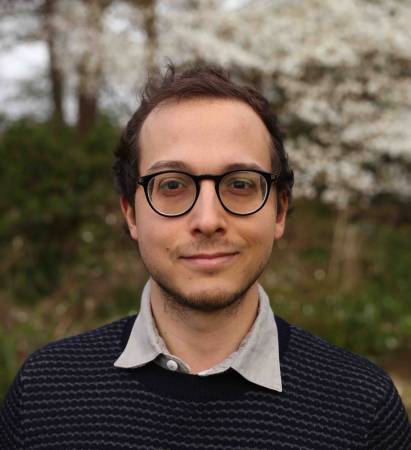
Abstract: Deep neural networks often lack generalization capabilities to accommodate changes in the input/output domain distributions and, therefore, are inherently limited by the restricted visual and semantic information contained in the original training set. In this talk, we argue the importance of the versatility of deep neural architectures and we explore it from various perspectives.
First, we briefly overview deep model adaptation to unseen visual domains with no ground truth annotations available and we observe how we can achieve domain invariance working at the input-, feature- or output- levels of the network.
Second, we address the ability of deep models to recognize novel semantic concepts without forgetting previously learned ones. We define continual semantic segmentation and we retain previous capabilities by distilling knowledge from the previous model, regularizing the latent space, or replacing samples of previous categories via generative networks or web-crawled images.
Finally, we discuss the recent federated learning paradigm to train deep architectures in a distributed setting exploiting only data available at decentralized clients and not shared with a central server. We define the general federated learning setup and we analyze its poor robustness to non-i.i.d. distribution of samples among clients. To mitigate this problem, we propose a naïve federated optimizer that is fair from the users’ perspective. Then, we introduce a new prototype-guided federated optimizer which has also been evaluated on federated semantic segmentation benchmarks.
Bio: Umberto Michieli is a Postdoctoral Researcher and Adjunct Professor at the University of Padua (Italy). His research interest lies at the intersection of some foundation AI problems, such as continual learning, federated learning, domain adaptation, applied to visual understanding tasks.
Homepage: https://umbertomichieli.github.io/
Sponsored in part by: Facebook Reality Labs Pittsburgh