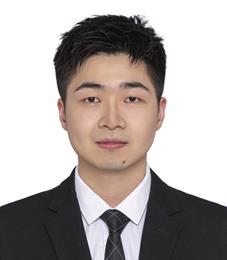
Abstract:
Detecting unseen instances based on multi-view templates is a challenging problem due to its open-world nature. Traditional methodologies, which primarily rely on 2D representations and matching techniques, are often inadequate in handling pose variations and occlusions. To solve this, we introduce VoxDet, a pioneer 3D geometry-aware framework that fully utilizes the strong 3D voxel representation and reliable voxel matching mechanism.
VoxDet first proposes a template voxel aggregation (TVA) module, effectively transforming multi-view 2D images into 3D voxel features. By leveraging associated camera poses, these features are aggregated into a compact 3D template voxel. In novel instance detection, this voxel representation demonstrates heightened resilience to occlusion and pose variations. We also find that a 3D reconstruction objective helps to pre-train the 2D-3D mapping in TVA. Second, to quickly align with the template voxel, VoxDet incorporates a Query Voxel Matching (QVM) module. The 2D queries are first converted into their voxel representation with the learned 2D-3D mapping. We find that since the 3D voxel representations encode the geometry, we can first estimate the relative rotation and then compare the aligned voxels, leading to improved accuracy and efficiency. Once trained, VoxDet does not need further tuning or optimization on novel instances.
Exhaustive experiments are conducted on the demanding LineMod-Occlusion, YCB-video, and the newly built RoboTools benchmarks, where VoxDet outperforms various 2D baselines remarkably with higher recall and faster speed.
Committee:
Prof. Sebastian Scherer (advisor)
Prof. Kris Kitani
Prof. Deva Ramanan
Zhiqiu Lin