
MSR Speaking Qualifier
July
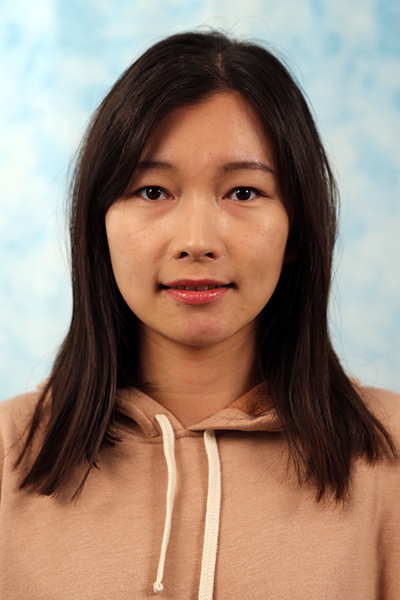
1:30 pm to 3:00 pm
NSH 3305
Title: Environment Generalization in Deep Reinforcement Learning
Abstract:
A key challenge in deep reinforcement learning (RL) is environment generalization: a policy trained to solve a task in one environment often fails to solve the same task in a slightly different test environment. In this work, we propose the “Environment-Probing” Interaction (EPI) policy, which allows the agent to probe a new environment to extract an implicit understanding of that environment’s behavior. Once this environment-specific information is obtained, it is used as an additional input to a task-specific policy that can now perform environment-conditioned actions to solve a task. To learn these EPI-policies, we present a reward function based on transition predictability. Specifically, a higher reward is given if the trajectory generated by the EPI-policy can be used to better predict transitions. We experimentally show that EPI-conditioned task-specific policies significantly outperform commonly used environment generalization methods on novel testing environments.
Committee:
Abhinav Gupta (advisor)
David Held
Lerrel Pinto