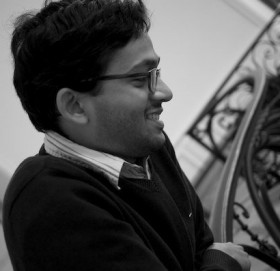
3:00 pm to 4:00 pm
Event Location: Newell Simon Hall 1507
Bio: Karteek Alahari is an Inria permanent researcher (chargé de recherche) since October 2015. He has been at Inria since 2010, initially as a postdoctoral fellow in the WILLOW team in Paris, and then on a starting research position in Grenoble since September 2013. Dr. Alahari’s PhD from Oxford Brookes University, UK, was on efficient inference and learning algorithms. His work as a postdoc focused on new models for scene understanding problems defined on videos. His current research interests are models for human pose estimation, semantic segmentation and object tracking, and weakly supervised learning.
Abstract: In this talk I will present the use of motion cues, in particular long-range temporal interactions among objects, for computer vision tasks such as video segmentation, object tracking, pose estimation and semantic segmentation. The first part of the talk will present a method to capture such interactions and to construct an intermediate-level video representation. We also use them for tracking objects, and develop a tracking-by-detection approach that exploits occlusion and motion reasoning. This reasoning is based on long-term trajectories, which are labelled as object or background tracks with an energy-based formulation. We then show the use of temporal constraints for estimating articulated human poses, which is cast as an optimization problem. We present a new approximate scheme to solve it, with two steps dedicated to pose estimation.
The second part of the talk presents the use of motion cues for semantic segmentation. Fully convolutional neural networks (FCNNs) have become the new state of the art for this task recently, but rely on a large number of images with strong pixel-level annotations. To address this, we present motion-CNN (M-CNN), a novel FCNN framework which incorporates motion cues and is learned from video-level weak annotations. Our learning scheme to train the network uses motion segments as soft constraints, thereby handling noisy motion information. We demonstrate that the performance of M-CNN learned with 150 weak video annotations is on par with state-of-the-art weakly-supervised methods trained with thousands of images.