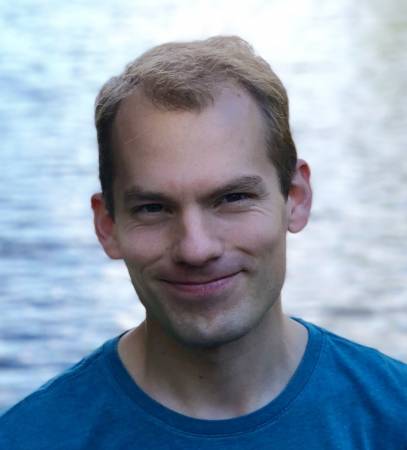
Abstract:
Contrastive learning organizes data by pulling together related items and pushing apart everything else. These methods have become very popular but it’s still not entirely clear when and why they work. I will share two ideas from our recent work. First, I will argue that contrastive learning is really about learning to forget. Different ways of setting up the objective result in different properties of the data being forgotten, and the trick is to find a way to forget everything except the really essential bits of information. Second, I will present a complementary argument from the perspective of geometry. I will show that contrastive objectives can be understood as optimizing two simple geometric properties of the data embedding, alignment and uniformity. Alignment refers to related items getting mapped to the same point in embedding space, and uniformity refers to the embedding having a uniform distribution. I will show that these two measures are highly predictive representation quality, and also can be directly optimized. I will end with some conjectures as to what else, beyond these properties, might be worth aiming for as we build the next generation of representation learning algorithms.
BIO:
Phillip Isola is an assistant professor in EECS at MIT studying computer vision, machine learning, and AI. He completed his Ph.D. in Brain & Cognitive Sciences at MIT, followed by a postdoc at UC Berkeley and a year at OpenAI. His current research focuses on how to make artificial intelligence more flexible, general, and adaptive.
Sponsored in part by: Facebook Reality Labs Pittsburgh