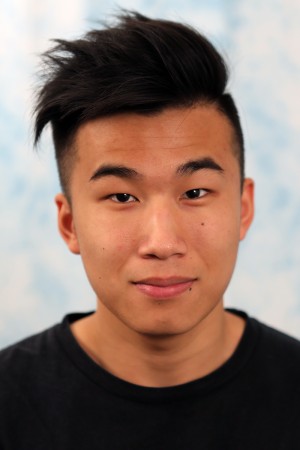
Carnegie Mellon University
3:30 pm to 4:30 pm
Location: https://cmu.zoom.us/j/96923127678?pwd=TWt3Zk5neFUzSlJWUjZEN2F6UVhudz09
Title: Representation Learning for Safe Autonomous Movement
Abstract:
Mobile robots have become an increasingly common presence in our homes and on our roads. To move safely within these shared spaces, autonomous agents must understand how other dynamic actors behave and how such behavior influences the navigability of the surrounding scene. Towards this goal, we propose two data-driven methods that learn representations from large, self-supervised corpora of data for 1) motion planning in hazardous, dynamic environments and 2) motion forecasting in autonomous driving settings.
The first method – active affordance learning (A2L) – advocates for a modular approach to autonomous navigation that combines learned spatial representations with traditional geometry-based maps and classical planning algorithms. By learning to predict a spatial affordance map (that encodes what parts of a scene are navigable) through active self-supervised experience gathering, we show that A2L is more sample efficient, generalizable, and interpretable than existing state-of-the-art reinforcement learning-based (RL) approaches.
The second method – what-if motion prediction (WIMP) – proposes a recurrent graph-based attentional approach for data-driven trajectory forecasting in autonomous driving settings. To the best of our knowledge, this is the first approach to demonstrate counterfactual motion forecasting based on topological queries such as map-based goals and social contexts. Using large-scale vehicle trajectory datasets, we demonstrate that WIMP outperforms previous learning-based methods and sets a new benchmark for prediction quality.
Committee:
Deva Ramanan (Advisor)
David Held
Adithya Murali