
MSR Thesis Defense
July
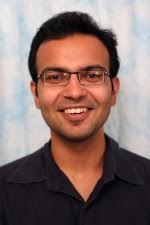
Carnegie Mellon University
3:00 pm to 4:00 pm
1305 Newell Simon Hall
Abstract
Thin objects, such as wires and power lines are one of the most challenging obstacles to detect and avoid for UAVs, and are a cause of numerous accidents each year. This thesis makes contributions in three areas of this domain: wire segmentation, reconstruction, and avoidance.
Pixelwise wire detection can be framed as a binary semantic segmentation task. Due to the lack of a labeled dataset for this task, we generate one by rendering photorealistic wires from synthetic models and compositing them into frames from publicly available flight videos. We show that dilated convolutional networks trained on this synthetic dataset in conjunction with a few real images perform with higher accuracy and speed on real-world data on a portable GPU as compared to multiple baselines.
Given the pixel-wise segmentations, we develop a method for 3D wire reconstruction. We propose a model-based multi-view algorithm, which employs a minimal parameterization of wires as catenary curves. We use a bundle adjustment-style framework to recover the model parameters using non-linear least squares optimization. In addition, we propose a model-free voxel grid method to reconstruct wires via a pose graph of disparity images, and briefly discuss the pros and cons of each method.
To close the sensing-planning loop for wire avoidance, we demonstrate a reactive, trajectory library-based planner coupled with our model-free reconstruction method in experiments with a real UAV. Finally, we propose a novel framework for long-range, deliberate avoidance. In current state-of-the-art sampling-based planners, collision checking is often the bottleneck and worst case asymptotic guarantees are the main focus. We propose a method to learn adaptive sampling distributions, such that the expected search effort is minimized for finite-time problems. Our non-stationary sampling heuristic leverages both the instantaneous search tree and the workspace environment, and shows significant runtime improvements in simulated experiments.
Speaker Bio
Ratnesh Madaan is an M.S. student in the Robotics Institute at Carnegie Mellon University, advised by Dr. Sebastian Scherer. He is broadly interested in perception and planning algorithms for UAVs. His current research interests lie in thin obstacle detection and mapping, and at the intersection of machine learning and traditional motion planning algorithms. Prior to CMU, Ratnesh received a B.Tech. in Mechanical Engineering from Indian Institute of Technology, Roorkee in 2015.