Face Recognition Across Illumination
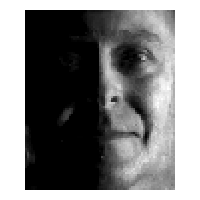
Recognizing people from their faces is an important task in many applications. Humans perform this task easily and robustly. We explore ways to develop an automatic face recognition system that can recognize faces from still images and videos.
A fully automated recognition system (from image capture to detection to recognition) is very useful in many areas. Applications include: visitor identification, building access control, security, suspect identification, digital video library archival/retrieval.
The task is difficult because the appearance of a face is dramatically altered by variations in illumination, facial expression, head pose, image size and quality, facial hair, cosmetics, accessories (such as eyeglasses), and age. To further compound the problem, we are often given only a few images of an individual from which to learn the distinguishing features, and then asked to recognize him in all possible situations.
The experience of other researchers show that appearance-based methods perform better than those based on geometry. Hence we will use appearance-based methods. After almost 30 years of face recognition research, we believe there are two important lessons that may be learned: (1) There is no single feature, or sets of features, that are truly invariant to all the variations mentioned above. Something that is invariant to illumination, for instance, is no longer invariant when pose is also changed. (2) Given more training images, almost any classification method perform better (smaller misclassification error). But in many applications, few training images are available.
The key idea then, is to artificially generate more training images, from the initial few that we started with. Then, from this enlarged set of training data, we learn an exemplar-based classifier. Intuitively, we can render all kinds of variations for each person: all combinations of pose, illumination, expression, etc. If we do this well, it is as if we had captured images of the person under all possible variations. We can now hope to recognize that individual under any situation. We demonstrate this idea by rendering faces under novel illumination, and show that it does indeed allow our classifier to cope with lighting changes.