Image Alignment
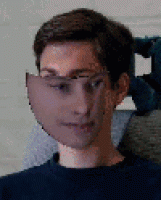
Parameterized Appearance Models (PAMs) (e.g. eigentracking, active appearance models, morphable models) use principal component analysis (PCA) to model the shape and appearance of objects in images. Given a new image with an unknown appearance/shape configuration, PAMs can detect and track the object by optimizing the model’s parameters that best match the image. While PAMs have numerous advantages for image alignment relative to alternative approaches, they suffer from three major limitations: First, PCA cannot model non-linear structure in the data. Second, learning PAMs requires precise manually labeled training data, which is an error prone and laborious task. Third, the search is prone to local minima problems, because of the bad generalization properties of PCA to unseen samples. This project aims to develop extensions of PCA that overcome these problems.
current head
current staff
current contact
past staff
- Xavier Boix Bosch
- Dan Casas
- Maria Teresa Ortin