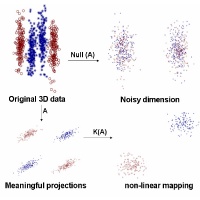
Learning Kernel Expansions for Image Classification
Project Head: Fernando De la Torre Frade
Kernel machines (e.g. SVM, KLDA) have shown state-of-the-art performance in several visual classification tasks. The classification performance of kernel machines greatly depends on the choice of kernels and its parameters. In this project, we propose a method to search over the space of parameterized kernels using a gradient-based method. Our method effectively learns a non-linear representation of the data useful for classification and simultaneously performs dimensionality reduction.
Displaying 1 Publications
current head
current contact
past staff
- Oriol Vinyals