PeepPredict
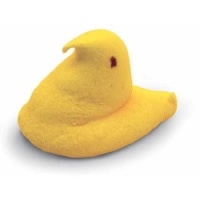
Predicting long-term and short-term behavior of people is a critical capability for robotic systems. The ability to understand behaviors increases the safety, efficiency and reliability of autonomous systems. These predictions must be produced in real-time and would ideally account for a wide variety of behavior types.
To this end, we are applying machine learning techniques to model and compute long-term and short-term trajectories of people in a variety of settings. We propose a novel structure for prediction that incorporates elements of planning to model purposeful behavior. The predictions that result are generic – in that they can be adapted to novel environments with little or no additional training data – and accurate – they beat the typical linear models.
current head
current contact
past staff
- Garratt Gallagher
- Kevin Peterson