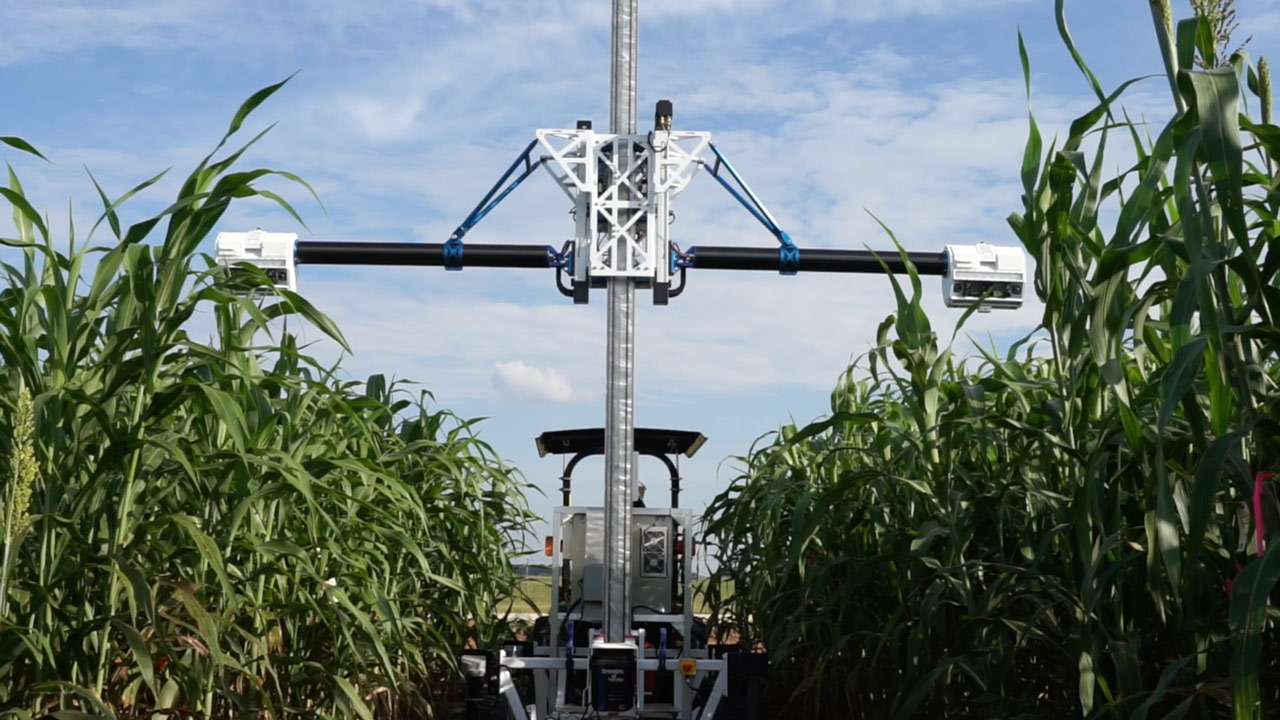
We are developing a robotic phenotyping systems for phenotyping crops for rapid breeding decisions. This system positions sensors within the canopy for measurements not observable from above or below. Machine learning and computer vision algorithms are then used to generate phenotyping data from the raw sensor data.
– Deployment of mobile system in between plots to maximize phenotypic data quality and quantity
– Creation of analysis approaches for genetic and breeding improvement
– Association of gene-traits that elucidate genetic pathways affecting yield in energy crops
– Application of technology in commercial sorghum breeding germplasm to produce faster rates of gains and new energy sorghum hybrids
Impact:
– Phenotyping system transforms breeding approaches to energy crops and functions for above and within canopy measurements, scalable to all energy and agronomic crops
– predictive estimates of traits (i.e., yield) allow indirect selection to increase performance
– Produce commercial quality bioenergy hybrids with improved yield, quality with increased rates of gain in future efforts.
This project also includes: Texas A&M, Clemson, University of Illionois, Purdue. And is funded by ARPA-E.
current head
current contact
past staff
- Barnabas Poczos
- Justin Scheifflee