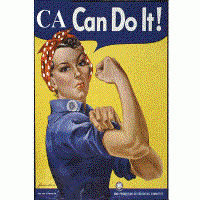
Over the last century, component analysis (CA) methods such as kernel principal component analysis, linear discriminant analysis, canonical correlation analysis, local linear projections, or spectral clustering have been extensively used as a feature extraction step for modeling, classification, image alignment, visualization, and clustering. CA techniques are appealing because many can be formulated as eigen-problems, offering great potential for learning linear and non-linear representations of the data without local minima. This project aims to find a least-squares unified framework to unify most CA methods. This unified framework will have several benefits: (1) provide a clean connection between many CA techniques, and a better understanding of the limitations and normalization factors, (2) yield efficient and robust numerical optimization approaches (e.g. avoid the small size sample problem), (3) a framework to extend many CA techniques.